Lead Researcher: Junshan Zhang
Research Synopsis: Minimum headway (distance) plays a critical role in vehicle safety in a transportation system. Despite significant effort, it remains challenging to set the safety distance in order to strike the right balance between the traffic throughput and collision risks.
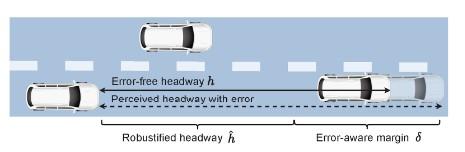
In the existing studies, most driving models set a safety distance based on the velocity of the following vehicle and the leading one, assuming perfect sensing. However, sensing errors are inevitable in practice, indicating that it is sensible to use a larger safety distance to mitigate collision risk, which nevertheless would reduce the total traffic throughput. Clearly, the determination of the safety distance hinges heavily on the sensing errors and risk tolerance models. Further, different from the traffic throughput which measures the total traffic, putting group safety above individual safety is unacceptable in general because no driver would choose to sacrifice his/her own safety to improve the group safety. That is to say, it would be too naive and perhaps unethical to simply maximize the group safety without paying attention to the individual safety.
To address these challenges, this research will develop a multi-objective optimization framework that can determine the Pareto front for the traffic throughput and the vehicle safety in a mixed-road environment. Specifically, to robustify vehicle safety we use an error-aware headway margin to provide resilience against sensing errors. As illustrated in Figure 1, the larger the error-aware headway margin is, the more safe the vehicle, which nevertheless would reduce the overall traffic throughput. We will formulate a bi-objective optimization problem to jointly maximize the traffic throughput and the vehicle safety with respect to the headway margin.